Understanding Human-Generated Data and Synthetic Data in AI Applications
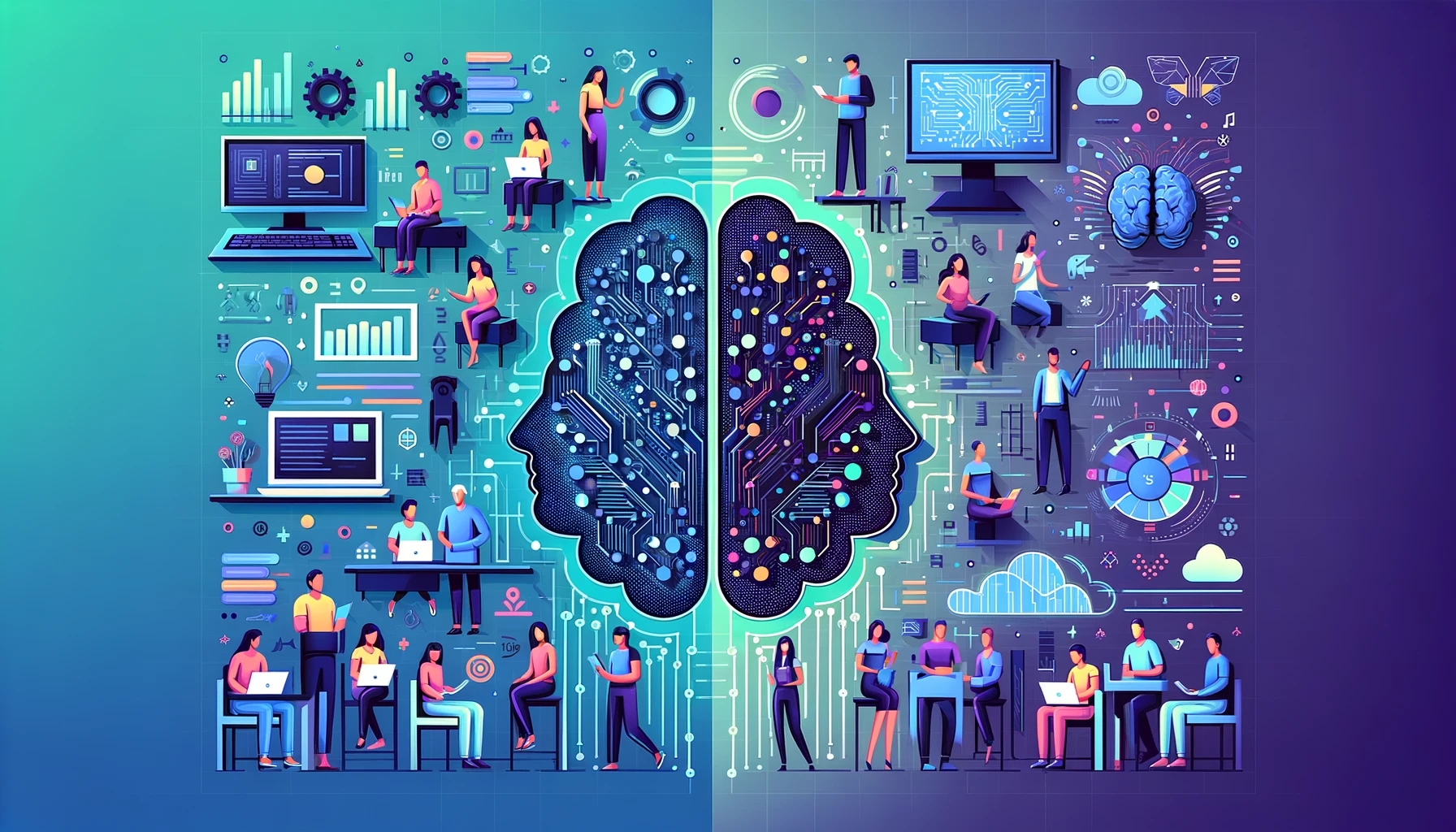
Data plays a crucial role in the advancement and efficacy of machine learning models within the ever-changing field of artificial intelligence (AI). There are two main categories of data that drive these advancements: human-generated data and synthetic data. Every type possesses distinct qualities, uses, advantages, and disadvantages. This article examines these distinctions, investigating the consequences for the advancement of AI and presenting concrete instances of their use and related challenges.
What is Human-Generated Data?
Human-generated data refers to information that is created or produced as a result of human actions or interactions. This encompasses information gathered from digital activities, such as engagements on social media platforms, online purchasing patterns, or search engine inquiries. It also includes data produced by users in different apps, such as text, photographs, and videos provided by individuals.
Benefits of Human-Generated Data:
- Authenticity: It accurately mirrors actual behaviours, preferences, and interactions, offering an authentic understanding of human dynamics.
- Richness: This data frequently encompasses a sophisticated comprehension of human context, emotions, and subtleties, which is highly beneficial for AI systems that require intricate decision-making capabilities.
Drawbacks of Human-Generated Data:
- Privacy Concerns: Gathering and utilising this data might give rise to substantial privacy concerns, particularly in the absence of explicit consent from the individuals whose data is being collected.
- Bias and Inaccuracy: Human data may possess inherent biases or flaws, which might manifest as the prejudices and faults of the individuals responsible for its creation.
What is Synthetic Data?
Synthetic data refers to artificially created information that imitates real data, but is not derived directly from authentic human interactions. It is commonly generated by algorithms or simulations to simulate different situations that AI may encounter, such as virtual surroundings for testing autonomous vehicles or generated photos for training facial recognition systems.
Benefits of Synthetic Data:
- Scalability: It has the capability to be manufactured in significant quantities as required, hence surpassing the constraints associated with limited or delicate real-world data.
- Privacy Preservation: Synthetic data mitigates numerous privacy risks by excluding actual individual data points.
Drawbacks of Synthetic Data:
- Lack of Realism: While synthetic data has shown some improvement, it may not fully capture the intricate subtleties of actual data, which could result in less efficient AI models.
- Dependence on Quality of Generation: The efficacy of synthetic data is contingent upon the algorithms employed for its generation, which may possess inherent flaws or biases.
Examples and Issues
Human-Generated Data Issues: Facebook’s Emotional Contagion Experiment:
In 2014, Facebook conducted a study on emotional contagion through social networks by altering the news feeds of 689,003 users. The project, deemed immoral, brought attention to significant issues with privacy and permission in the use of human-generated data.
Synthetic Data Issues: Autonomous Vehicle Testing:
Waymo and Tesla utilise fake scenarios to train their driving algorithms. Nevertheless, there have been occurrences in which these vehicles encountered difficulties in real-life situations due to the synthetic data’s inability to accurately represent unpredictable human behaviour or rare events.
Conclusion
Both data supplied by humans and data generated synthetically are essential in enhancing the capabilities of artificial intelligence. Human-generated data provides valuable insights into genuine human behaviours and interactions, enhancing the relevance and adaptability of AI systems to real-world circumstances. Conversely, synthetic data provides a privacy-compliant and scalable alternative that is extremely beneficial in instances when there is a shortage of genuine data or where the real data is sensitive.
Nevertheless, the decision about whether to use human-generated data, synthetic data, or a combination of both should be determined based on the specific needs of the AI application, ethical factors, and the potential consequences for society. To design strong, fair, and efficient AI systems, developers can efficiently utilise the benefits of each form of data by comprehending and addressing their related shortcomings.