The Importance of Good Data in AI Training: Why Quality Matters
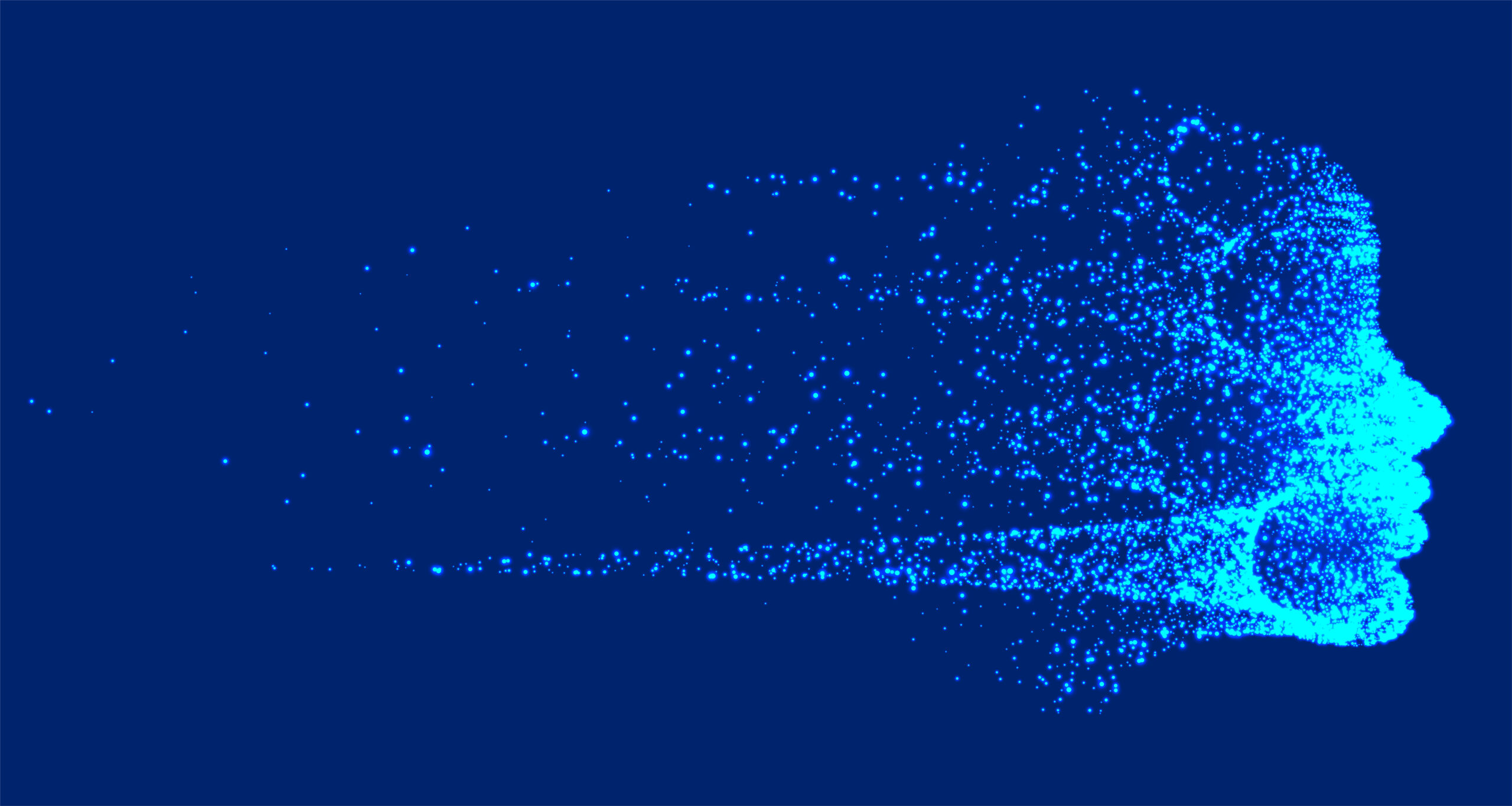
Introduction
Rapid developments in AI technology have resulted in its widespread adoption across many sectors, from healthcare to the financial sector. However, the quality of data used during training is crucial to developing an efficient and effective AI system. In this post, we’ll go over why it’s so important to guarantee data quality when creating AI solutions, the crucial role that good data plays in training, and the potential pitfalls that bad data can cause.
The Role of Data in AI Training
Data is the energy source for AI programmes. To equip AI models with the ability to predict and make decisions, machine learning algorithms sift through massive amounts of data during the training process. Artificial intelligence (AI) systems are only as good as the data they are trained on.
Good Data vs. Bad Data
The best data is complete, current, applicable, and objective, covering a wide variety of conditions and inputs. This makes sure AI systems can deal with complex problems and produce reliable outcomes in the real world. On the flip side, a poorly trained AI system is the result of inaccurate, unreliable, irrelevant, or biased data.
Why Good Data Matters
- AI systems that have been properly trained on high-quality data are able to make more precise and trustworthy predictions and judgments. This improves performance and makes users happier.
- Users are more likely to trust AI systems and their output and recommendations when those models are constructed using high-quality data. In fields like healthcare and finance, where AI-powered decisions can have significant impacts on people’s lives, trust is especially important.
- Risk of biased AI systems is reduced when a diverse and representative dataset is used. Legal, ethical, and public-image problems can all arise when biased AI is used to perpetuate unfair and stereotypical practises.
- Cost-savings and quicker rollouts are the result of developers making the most of their computing resources during the training of AI systems with high-quality data.
The Consequences of Bad Data
- Prediction and decision errors caused by AI systems that were taught with inaccurate data have the potential to have far-reaching effects, particularly in sectors like healthcare, finance, and transportation.
- When users’ faith in AI systems declines because the technology consistently disappoints them, they are less likely to adopt it and are more likely to pass up opportunities.
- Unfair treatment of some groups and possible legal and ethical issues may result from AI systems that have been trained on bad data, which can perpetuate and even amplify existing biases.
- Training AI systems on poor data wastes time and money by requiring more processing power than is necessary.
A few examples of where data quality can have a significant impact:
Healthcare AI System for Diagnosing Diseases
Good Data Scenario: A large, diverse, and representative dataset of medical images and patient information is used to train an AI system, which may include patients of varying ages, races, and genders. The dataset has been carefully curated to include accurate diagnoses and pertinent data. Consequently, the AI system’s diagnostic accuracy across diseases is high, leading to better patient outcomes and fewer incorrect diagnoses.
Bad Data Scenario: An AI system is trained to diagnose disease by analysing data from a small subset of patients, typically men of a certain age and background. Misdiagnoses and other errors can also be found in the dataset. The resulting AI system has a high propensity for making incorrect diagnoses in female patients, patients of different ages, and patients of different races. Incorrect treatments are administered, putting patients at risk and damaging faith in the AI system.
Example 2: AI-powered Loan Approval System for a Bank
Good Data Scenario: A bank has adopted AI technology to speed up the loan approval process. The dataset used to train the system is exhaustive and representative of real-world loan applicants across a wide range of demographics and financial circumstances. This makes sure the AI system approves more qualified applicants and reduces risk for the bank by making accurate assessments of their creditworthiness.
Bad Data Scenario: The same bank’s AI is trained on a dataset that is skewed toward applicants from affluent areas and lacks sufficient data on applicants with lower credit scores or diverse backgrounds. As a result, the AI is prejudiced and turns down qualified borrowers from low-income areas, contributing to the cycle of poverty.
Example 3: AI-based Job Applicant Screening Tool
Good Data Scenario: To narrow down the pool of potential new hires, one company has turned to an AI-powered applicant screening tool. The software is educated on a large sample of resumes and applications from a wide range of fields, job descriptions, and applicant backgrounds. The AI system can efficiently and fairly identify qualified candidates based on their skills, experience, and qualifications.
Bad Data Scenario: The company uses a dataset consisting primarily of resumes from applicants of a certain gender, age range, or educational background to train its artificial intelligence applicant screening tool. The resulting AI is discriminatory, giving preference to candidates who meet the strict demographic requirements while unfairly excluding qualified applicants from more diverse backgrounds. This could cause legal problems, harm the company’s reputation, and reduce the diversity of its employees.
Conclusion
The efficiency, precision, and credibility of AI systems are heavily dependent on the quality of the data used in their training. Improving AI performance and user satisfaction can be achieved by reducing the likelihood of errors, biases, and poor decision-making during model training. To realise the full potential of this game-changing technology, developers and organisations must place a premium on data quality during the AI development process.