Harnessing Artificial Intelligence to Eliminate Bias from Data
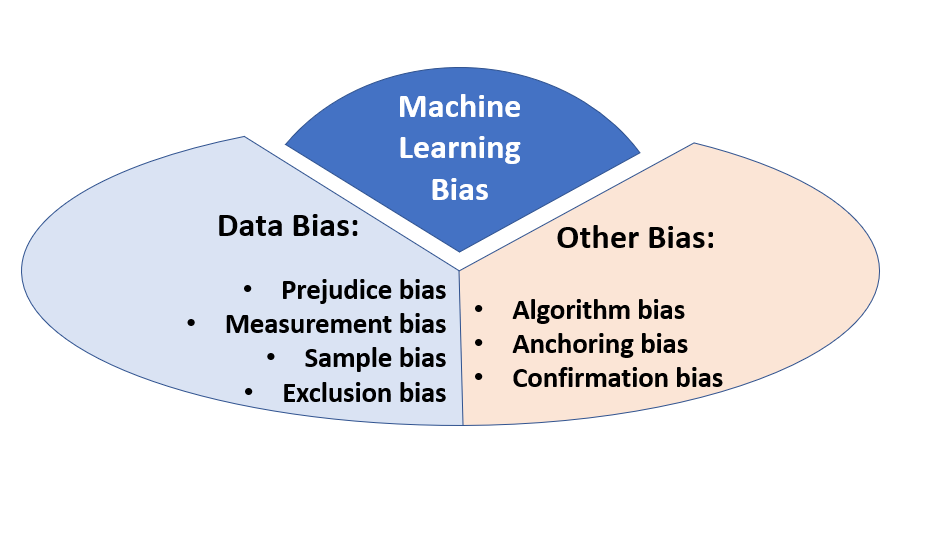
Artificial Intelligence (AI) has disrupted various domains by providing unparalleled functionalities in the realms of data processing, analysis, and decision-making. Nevertheless, due to their reliance on historical data for learning, AI systems are vulnerable to acquiring and propagating the biases that are inherent in said data. This can give rise to inequitable and biassed results in a multitude of contexts, encompassing loan approvals and employment procedures. In light of this difficulty, scholars and practitioners are investigating novel methodologies to utilise AI in order to detect and alleviate bias in pre-existing datasets, thus promoting the development of AI applications that are more equitable. This article explores the ways in which artificial intelligence (AI) can be employed to automatically eliminate bias from pre-existing AI data. It emphasises recent developments and methodologies in this field.
Identifying Bias through AI
Identifying and quantifying bias in AI datasets is the initial step in mitigating it. It is possible to train AI algorithms, specifically those utilising machine learning (ML), to identify patterns and anomalies in data that could potentially signify bias. An illustration of this is the utilisation of natural language processing (NLP) algorithms to detect gender or racial stereotypes in text data; this technique has been implemented in a number of studies attempting to eliminate bias from AI-generated content [1].
Fairness Metrics
For the purpose of systematically addressing bias, researchers have developed a variety of fairness metrics for use by AI systems in assessing their own decisions. The application of quantitative measures, including demographic parity, equality of opportunity, and predictive parity, facilitates the evaluation of fairness among distinct social groups. By integrating these metrics into AI models, programmers can guarantee that their algorithms strive for equitable results in addition to precision.
Bias Mitigation Algorithms
After bias has been identified, procedures to mitigate it must be implemented. In pursuit of this objective, numerous algorithms have been devised to function at distinct phases of the process of developing AI models:
- Pre-processing Techniques: These techniques require the training data to be altered prior to its use in training an AI model. Methods such as resampling to achieve a more balanced representation of classes or feature transformation to eliminate biassed associations can be implemented to safeguard against the data perpetuating pre-existing inequalities [2].
- In-processing Techniques: These methodologies encompass the direct incorporation of fairness constraints during the process of training the model. By simultaneously maximising accuracy and fairness, these methodologies can direct the model towards acquiring representations that are devoid of bias. Incorporating regularisation terms that penalise bias in the model’s predictions is one example.
- Post-processing Techniques: Once a model has been trained, its predictions can be adjusted using post-processing techniques to ensure fairness. This may entail adjusting the decision threshold to ensure that all groups attain equivalent results, or recalibrating the model’s outputs in accordance with fairness standards.
Case Studies and Applications
The application of AI to reduce bias is exemplified by the domain of facial recognition technology. Research has indicated that certain facial recognition systems demonstrate bias, as evidenced by their diminished accuracy when applied to specific demographic groups. Sophisticated algorithms and more equitable datasets have been devised by researchers to rectify these inequities, resulting in enhanced precision and impartiality among heterogeneous populations.
Ethical Considerations and Challenges
Although the potential of artificial intelligence to reduce bias in datasets is encouraging, it also gives rise to ethical concerns. Regarding the definition of fairness, there exists considerable subjectivity and variation among cultures and contexts. Therefore, considering ethical principles and the possibility of unintended consequences is crucial when implementing AI for bias mitigation. Furthermore, it is imperative to maintain transparency and accountability in AI systems in order to foster confidence and guarantee their responsible implementation.
Conclusion
The implementation of AI for the automated identification and reduction of bias in datasets signifies a substantial advancement towards the development of AI systems that are equitable and fair. Developers can mitigate the potential for persisting inequalities by implementing fairness metrics, bias mitigation algorithms, and ongoing evaluation and refinement of AI models. However, due to the complexity of bias and the ethical ramifications of AI interventions, their implementation requires caution and deliberation. Ongoing collaboration among technologists, ethicists, and policymakers will be imperative as the field develops in order to effectively leverage the advantages of AI while mitigating its potential drawbacks.