The Shift from Proprietary NVIDIA AI Solutions to Open Source Frameworks: Why Enterprises are Making the Move
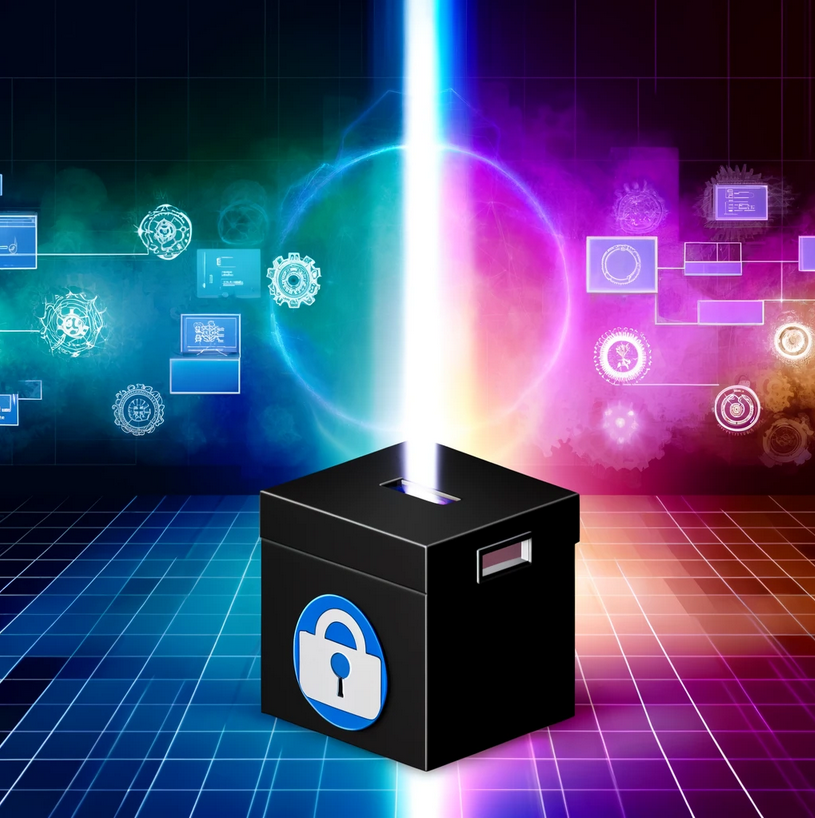
In recent years, artificial intelligence (AI) has emerged as a fundamental element of innovation across various industries. Businesses, ranging from startups to multinational businesses, have been significantly investing in AI to enhance efficiencies, get insights, and develop new goods and services. Historically, numerous AI workloads have been driven by NVIDIA’s proprietary technologies, which have become emblematic of high-performance AI processing. Nonetheless, a notable transition is occurring: an increasing number of organisations are migrating their AI workloads from proprietary NVIDIA solutions to more open-source frameworks. This paper investigates the motivations for this trend, analyses the advantages of open-source AI frameworks, and underscores notable instances of this shift.
The Allure of Proprietary Solutions: Why NVIDIA Was the Go-To for AI
NVIDIA has consistently led in the development of AI hardware and software. The GPUs are specifically optimised for deep learning and other AI-related applications, rendering them the preferred hardware for numerous AI researchers and organisations. NVIDIA has created a collection of proprietary software tools, such as CUDA and cuDNN, designed to optimise performance from its GPUs in conjunction with this technology.
The amalgamation of hardware and software has established a robust environment that facilitates superior performance and scalability, which is why numerous organisations have always favoured NVIDIA for their AI tasks. The company’s exclusive solutions provide:
- Enhanced Performance: NVIDIA’s tools are designed to maximise the capabilities of its GPUs, delivering unmatched speed and efficiency in training and inference.
- Support and Reliability: NVIDIA provides a comprehensive single vendor solution, including substantial support and consistent upgrades, thereby guaranteeing organisations a stable and dependable platform for developing their AI applications.
- Wide Adoption and Community Support: NVIDIA’s AI tools have a substantial user community, resulting in a comprehensive ecosystem that offers abundant knowledge, tutorials, and third-party integrations.
The Shift to Open Source: Why Enterprises are Moving Away from Proprietary Solutions
Notwithstanding the benefits provided by NVIDIA, an increasing number of organisations are transitioning to open-source AI frameworks. This tendency is influenced by multiple factors:
1. Cost Considerations
NVIDIA’s proprietary solutions might be costly for both hardware and software. Licensing fees, in conjunction with the expense of premium GPUs, can rapidly accumulate, particularly for large-scale organisations. Conversely, open-source frameworks typically incur no license fees and are compatible with a broader array of hardware, including cost-effective alternatives such as CPUs and non-NVIDIA GPUs. This adaptability can result in considerable cost reductions.
2. Avoiding Vendor Lock-In
Vendor lock-in poses a considerable risk for organisations dependent on proprietary systems. Transitioning to a different supplier or technology can be expensive and labour-intensive once an organisation has established its AI infrastructure with a particular vendor. Open-source frameworks provide enhanced flexibility, enabling organisations to circumvent dependency on a sole vendor. This autonomy allows organisations to swiftly adjust to emerging technologies and mitigate possible disruptions should a vendor’s technology become outdated or prohibitively costly.
3. Customisation and Flexibility
Open-source AI frameworks grant access to the source code, enabling organisations to alter and tailor the program to suit their particular requirements. This degree of customisation is frequently unattainable with proprietary solutions, which tend to be more inflexible in their execution. The capacity to customise AI tools for particular applications is a considerable benefit for organisations with distinct needs.
4. Innovation and Community Support
The open-source community fosters innovation, with several developers contributing to the swift advancement of AI tools and frameworks. This collaborative environment frequently results in accelerated development cycles and the implementation of innovative features that may require more time to emerge in proprietary systems. Moreover, the extensive collection of community resources, such as forums, documentation, and plugins, facilitates the adoption and integration of open-source solutions by organisations.
Examples of Open Source AI Frameworks
Numerous open-source AI frameworks are emerging as credible alternatives to NVIDIA’s proprietary technologies. Notable examples include:

- OpenVINO™ (Open Visual Inference and Neural Network Optimization): OpenVINO, developed by Intel, is intended to optimise and implement AI inference across several hardware platforms, including CPUs, GPUs, and FPGAs. It offers a strong alternative to NVIDIA’s TensorRT, especially for organisations aiming to optimise the performance of their non-NVIDIA technology.
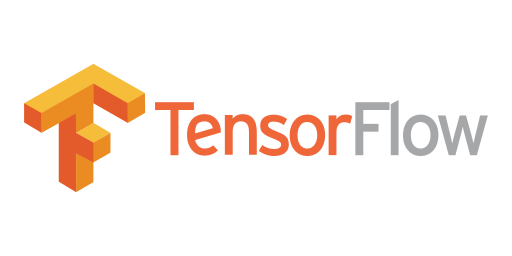
- TensorFlow: Despite being initially optimised for NVIDIA GPUs, TensorFlow’s open-source framework and extensive community backing have resulted in several optimisations for various hardware platforms. TensorFlow has been fundamental to numerous AI applications and is compatible with diverse processing units, rendering it a flexible option for businesses.
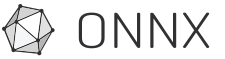
- ONNX (Open Neural Network Exchange): ONNX is an open-source standard for artificial intelligence models that facilitates their transfer across various frameworks and hardware platforms. Originally created by Microsoft and Facebook, ONNX has established itself as a standard for guaranteeing the interoperability of AI models across diverse platforms.
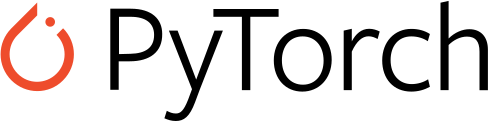
- PyTorch: PyTorch, a prevalent open-source deep learning framework, has attained popularity because to its user-friendliness, adaptability, and robust community support. Although it was originally optimised for NVIDIA GPUs, its open-source design facilitates wider device compatibility.
Real-World Examples of the Shift
Numerous prominent companies have been shifting from NVIDIA’s proprietary solutions to open-source AI frameworks, motivated by the want for enhanced flexibility, cost efficiency, and the desire to evade vendor lock-in. Several prominent instances encompass:
- Microsoft: Despite being a partner of NVIDIA, Microsoft has progressively invested in open-source frameworks such as ONNX, which it co-developed. ONNX enables Microsoft to retain adaptability in deploying AI models across its Azure cloud platform, accommodating various hardware, including alternatives to NVIDIA.
- Amazon Web Services (AWS): AWS has included open-source frameworks like TensorFlow and PyTorch into its cloud services, enabling clients to execute AI workloads on many hardware alternatives beyond NVIDIA GPUs. This diversity allows AWS to provide more customised solutions to its clients based on their particular requirements.
Conclusion: The Future of AI Workloads
The transition from proprietary NVIDIA AI solutions to open-source frameworks signifies a wider trend towards adaptability, cost-effectiveness, and innovation in the enterprise AI domain. Although NVIDIA’s solutions have a prominent position in the industry, the benefits provided by open-source alternatives are becoming increasingly difficult to overlook. As organisations expand their AI initiatives and investigate novel applications, the attractiveness of open-source frameworks such as OpenVINO, PyTorch, and ONNX is expected to increase.
In this changing environment, organisations must meticulously evaluate their AI strategy, weighing the advantages of proprietary and open-source technologies to effectively address their requirements. The future of AI will probably be characterised by a hybrid approach, wherein the flexibility and creativity of open-source frameworks enhance the efficacy and dependability of proprietary technologies.
The transformation is already under progress, and as other firms adopt this change, the AI ecosystem will further develop, promoting enhanced collaboration, innovation, and diversity in AI applications across many industries.