Using AI and ML to Make Storage Systems More Powerful: A Tale of Two Architectures
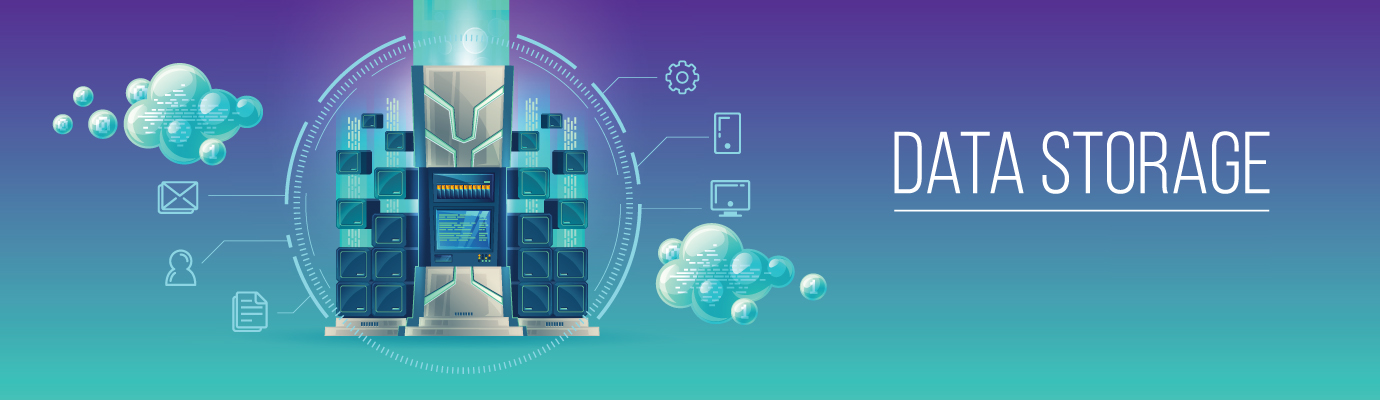
Introduction
As a result of the digital revolution, there has been an unprecedented need for reliable and scalable data storage options. There are primarily two types of storage architectures in use today: legacy storage systems (such as DAS, NAS, and SAN) and software-defined storage (SDS). While both have their benefits, they must contend with the increasing difficulty of effectively managing data volumes. Game-changing technologies like Artificial Intelligence (AI) and Machine Learning (ML) can turbocharge both architectures, boosting their performance, efficiency, and adaptability.
Leveraging AI and ML in Conventional Data Storage Systems
For decades, conventional storage architectures have provided dependable storage options for a wide range of uses. But the ever-increasing data volumes require a smarter strategy for storage management.
- Intelligent Data Tiering and Resource Management: By analysing data access patterns and performance requirements in real time, AI and ML can optimise data tiering by automatically migrating data between different types of storage. To further improve resource allocation and prevent wasteful over- or under-provisioning, these technologies can also analyse historical patterns of resource utilisation.
- Proactive Maintenance and Failure Prediction: Every storage system will eventually experience hardware failure. By keeping tabs on the condition of a system and flagging any warning signs or impending problems, AI and ML can facilitate preventative maintenance and cut down on downtime.
- Advanced Data Deduplication and Compression: Improved storage efficiency can be achieved through data deduplication and compression with the help of AI and ML algorithms that can more accurately identify redundant data.
- Security and Anomaly Detection: An additional line of defence against data breaches can be implemented with the help of AI and ML by monitoring for anomalies and potential threats in real time.
Leveraging AI and ML in Software Defined Storage
With its software-centric design, SDS improves upon traditional data storage in terms of scalability, adaptability, and economy. However, there are still obstacles to overcome when dealing with massive amounts of data in SDS. Integration of AI and ML can help get past these problems.
- Intelligent Data Placement and Migration: Data placement can be optimised by AI and ML considering access patterns, performance requirements, and available resources. The end result is an adaptive storage system that makes the most of its available resources and operates at peak efficiency.
- Predictive Analytics for Performance Management: With the help of AI and ML, we can analyse past results, identify trends, and plan for the future. SDS systems can better adjust resources and foresee storage needs because of their forward-thinking nature.
- Anomaly Detection and Data Protection: AI and ML can keep a constant eye on a storage system to look for any signs of trouble. Organizations can prevent further damage and data loss if they catch problems early.
- Self-Healing and Autonomous Management: With the help of AI and ML, SDS systems can diagnose and fix problems on their own, cutting down on management time and downtime while freeing up IT resources for more strategic endeavours.
Conclusion
No matter the storage architecture, adding AI and ML technologies can greatly improve the system’s speed, efficiency, and flexibility. When it comes to intelligent resource management, failure prediction, security improvements, and data placement optimization, AI and ML are changing the game for both traditional and software-defined storage systems. We can’t survive in today’s data-driven world without incorporating AI and ML into our storage solutions.