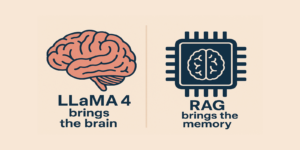
Not long ago, I wrote about why Retrieval-Augmented Generation (RAG) is such a pivotal architecture in modern AI workflows, particularly when compared to fine-tuning and training from scratch. The core argument was simple: RAG enables models to stay up-to-date, grounded, and efficient without massive retraining costs. It was (and still is) a pragmatic solution to […]